Krishna Amin
Krishna is a PhD student at the Digital Environment Research Institute (DERI) in the AI in Drug Discovery programme. Before joining the programme, he was at the University of Cambridge, from where he holds an MSci. (Hons) in Systems Biology and B.A. (Hons) in Natural Sciences (specialising in Biochemistry).
Krishna has a strong interest in using multidimensional data to build and model complex networks in the human body, to better understand complex disease and identify means to improve quality of life. His project uses multi-omics data from volunteers around the world to build patient-derived networks of age-related diseases (ARDs). These data are linked with volunteers’ medical histories, providing valuable personal insight and data dimensionality. He aims to apply these networks to uncover mechanisms of ARDs, facilitate drug target discovery and patient stratification, and predict ARD development in individuals.
In his free time, Krishna plays tennis for Queen Mary, plays the piano and viola, and practices his photography. He has also recently picked up archery and kendo at the University.
Project Title: Multi-Omics systems biology modelling
Supervisors: James O'Reilly - Data Scientist at Recursion | Damian Smedley - Professor in Computational Genomics, The William Harvey Research Institute, QMUL
William Dee

William is a PhD candidate focusing on the detection of druggable features from high-content imaging data using Artificial Intelligence. He has completed Master’s degrees in both Data Science and Bioinformatics and is enthusiastic about applying cutting-edge machine learning approaches to biological data. His prior work includes research in the field of antimicrobial peptide prediction leveraging large-scale language models, and utilizing generative models to create synthetic thyroid histopathology data.
Project Title: Detection of druggable features from high content imaging data
Supervisors: Otto Morris - Senior Biological Data Scientist at Recursion | Greg Slabaugh - Director of the Digital Environment Research Institute (DERI) & Prof of Computer Vision and AI, QMUL
Stylianos Iliadis

Stylianos has a degree in Pharmacy (Aristotle University of Thessaloniki - Greece) and an MSc in Medicinal Chemistry (University of Copenhagen - Denmark). During his undergraduate studies, he worked on wet-lab projects, but after realising the impact of computational methods in Drug Design, he undertook a Computational Drug Design MSc project. In this project, he worked on the design and discovery of novel ligands for orphan GPCRs by applying related computational methods such as MD simulations and molecular docking.
Stylianos' research interests lie in the Computational Drug Design against GPCRs. GPCRs have a central role in cellular communication which makes them involved in numerous pathological situations. Due to their complexity, the application of computational methods is necessary to study them effectively and eventually target them for reverting pathology. The efficient design of ligands can take place via the integration of chemical, biological, and structural information, which allows for making better decisions during the drug design process.
Recent advancements in GPCRs structural biology have provided an unprecedented number of GPCR structures, thereby revealing details on GPCR structures, based on which structure-based drug design (SBDD) methods can be applied. Additionally, chemogenomics and structural bioinformatics methods as well as drug design-relevant data such as ligand molecular and structural properties can also be harnessed and integrated to effectively design novel ligands. During his AIDD project, Stylianos will work on the development and application of machine learning-augmented structural bioinformatics and chemogenomics methods for guiding SBDD efforts.
Project: Structural Bioinformatics and chemogenomics approaches
Supervisors: Pierre Matricon – Senior Scientist at Nxera | Arianna Fornili - Senior Lecturer in Computational Chemistry, School of Physical and Chemical Sciences, QMUL
Yuan Liang
Hi there! I am an Artificial Intelligence and drug discovery intersection Ph.D. student, interested in the application of Natural Language Processing algorithms in the drug discovery process. I am originally from China and got my Bachelor's degree here in Informational and Computation Science (Computing Mathematics). Then, I went to the University of Edinburgh to study High-Performance Computing with Data Science in 2016. After graduation, I went back to China and became an NLP engineer. Till now, I have worked for more than five years and applied NLP algorithms in different business scenarios, such as advertisement, news, and chatbot. In my free time, I like to play with my cat, play pc games or switch and do some sports.
I am so glad to have an opportunity to go back to school and do an interesting project, called next generation text mining in drug discovery. The project aims to extract useful information from natural language format medical documents, such as small molecular and their targets, and use such information to identify and build specific signatures for drug-gene associations, chemical and biological toxicity and even adverse drug effects.
Project Title: Next generation text mining in drug discovery
Supervisors: Roonak Rezvani - Data Scientist at Recursion | Massimo Poesio - Professor of Computational Linguistics, School of Electrical Engineering and Computer Science, QMUL
Lewis Palmer

The drug discovery process is costly and time-consuming, while also suffering from high rates of attrition. Failure of drugs to pass stringent clinical trial criteria is often caused by safety concerns or lack of greater efficacy than established therapies. This project seeks to leverage the wealth of publicly available data to discover novel drug targets, while also prioritising those least likely to have off-target effects or lack sufficient efficacy. This will be achieved by combining elements of systems and structural biology with state-of-the-art machine learning techniques.
Project Title: Prioritising Drug Targets in non-canonical pathways from multi-omic data
Supervisors: Kirill Shkura - Systems Biologist and Data Scientist, MSD | Prof Conrad Bessant - Professor of Bioinformatics, School of Biological and Behavioural Sciences, QMUL
Aaron Wenteler
Aaron Wenteler is a PhD student on the AI for Drug Discovery Programme, and also part of the Queen Mary’s Centre for Translational Bioinformatics where he is researching ways to improve drug discovery by leveraging AI. He has previously completed his Bachelor’s and Master’s studies in Nanobiology at the Delft University of Technology in The Netherlands. Here, he was taught to look at biological problems through the lens of an engineer as the Nanobiology study programme was established in order to train researchers that can operate at the intersection between Physics, Computer Science, and Biology. In his current position, he is using AI to extract valuable information from the vast amount of available biological data in order to inform drug discovery.
Project Title: Using AI to investigate and modulate gene function in patient populations and biobanks
Supervisors: Dr Wei Wei -Senior Scientist at MSD | Prof Mike Barnes - Professor of Bioinformatics and Director of the Centre for Translational Bioinformatics, The William Harvey Research Institute, QMUL
Wei Dai
Wei (Dave) Dai is a PhD student in the AI for drug discovery programme at DERI. He completed a bachelor's de
gree in Chemistry at University of Bristol and a master’s degree in Digital Chemistry at Imperial College London. In previous projects, Wei has focused on using machine learning tools to solve bio/cheminformatics problems, such as blood-brain barrier penetration prediction and nanopore data analysis.
Wei has a great interest in the practical application of machine learning in the drug discovery process. His project uses protein-ligand interaction data to develop several machine learning models which enable the design of efficacious therapeutic compounds targeting GPCRs. Wei will develop, evaluate, and optimize new methods, and his ultimate goal is to apply the developed models to the GPCR drug development cycle.
Project Title: Cheminformatics and Machine Learning approaches for GPCR Computer-Aided Drug Design
Supervisors: Jonathan Tyzack – Lead Scientist, Nxera Pharma | Arianna Fornili - Senior Lecturer in Computational Chemistry, School of Physical and Chemical Sciences, QMUL
Bernhard Finke
Bernhard is a PhD student in the 2023 AI for Drug Discovery cohort. Previously he completed a BSc in Mathemati
cs and Statistics at the University of Essex and an MSc in Artificial Intelligence at the University of Edinburgh, where he developed an interest in applying AI and bioinformatics to biomedical research.
Now based primarily at the Centre for Cancer Genomics & Computational Biology at Barts Cancer Institute, his project involves integrating genomic and digital pathology data to predict metastasis in cutaneous squamous cell carcinoma (cSCC) using AI. cSCC is the second most common type of skin cancer worldwide and metastasis is associated with significantly worse outcomes. Currently prediction of cSCC metastasis is highly inaccurate, so a well-designed and interpretable model will allow clinicians to effectively target appropriate interventions.
Project Title: Predicting metastasis and drug response in skin cancer using multimodal deep learning
Supervisors: Jun Wang - Senior Lecturer, Barts Cancer Institute, QMUL | Catherine Harwood - Clinical Professor of Dermato-Oncology, Blizard Institute, QMUL
Nasim Mohamed Ismail
Nasim is a PhD student in the AI for Drug Discovery Programme. Before joining the programme, he completed a BSc in Pharmacology and Innovative Therapeutics and an MSc in Bioinformatics at Queen Mary University of London.
His project involves exploring AI data modelling and fusion methods that effectively integrate multi-omics data as well as other modalities such as imaging data for holistic prediction, using emerging high-dimensionality human datasets for neurodegenerative diseases such as ALS, Alzheimer's disease and Parkinson’s disease, with the aim of applying novel multi-modal integration methods in the discovery of new targets and biomarkers.
Project Title: Multimodal AI for Multi-omics Data Integration
Supervisors: Kirill Shkura - Systems Biologist and Data Scientist, MSD | Greg Slabaugh - Professor of Computer Vision, and Director of the Digital Environment Research Institute, QMUL | James Timmons - Reader in Translational Bioinformatics, William Harvey Research Institute, QMUL
Caitlin Mellor
Caitlin is a PhD student in the AI for Drug Discovery Programme based at DERI. She completed a BSc in Biomedicine at the University of East Anglia, followed by a PG Cert in Bioinformatics at the University of Birmingham.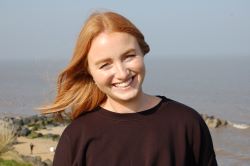
Her project involves logical modelling to combine existing knowledge about CDKs with synthetic interaction data to develop an experimentally validated model for phospho-regulation of cell cycle control. The project aims to establish a framework which can be applied to proteins globally, supporting the identification of novel therapeutic interventions for cell cycle driven diseases.
Project Title: Modelling the phospho-regulation of cell cycle control
Supervisors: Peter Thorpe - Senior Lecturer in Biochemistry, School for Biological and Behavioural Sciences, QMUL | Radu Zabet - Senior Lecturer in Computational Biology, the Blizard Institute, QMUL
Martina Occhetta
Martina is a doctoral candidate in the AI for Drug Discovery programme. She holds an MPhil in Computational Biol
ogy from the University of Cambridge and a BSc in Biochemistry from Imperial College London. Martina's current work revolves around the development and refinement of robust explainable AI techniques to mine large multi-omics perturbation datasets.
Her primary interest lies in devising supervised learning models that can accurately predict how individual genes and proteins respond to chemical perturbations. Through this work, Martina aims to identify potential new drug targets, paving the way for advancements in the development of therapeutic strategies.
Project Title: Target identification from multi-omics data using systems biology and machine-learning approaches
Supervisors: Mani Mudaliar - Target Analyst Director at Recursion | Conrad Bessant - Professor of Bioinformatics, School of Biological and Behavioural Sciences, QMUL
Jacky Siu
Jacky is a part of the second cohort within the UKRI-AIDD programme, with strong research interest in explainable artificia
l intelligence and its application on biological data. He has completed his MSc in Bioinformatics (University of Wageningen and Research) and will be working on automated target and biomarker selections using natural language processing-based methods, to decipher the unbiased decisions from complex network of information relationships. His prior work includes research in the field of population genetics, performing demographic inference of Sub-Saharan Africa mosquito populations with generative adversarial networks.
Project Title: Target/Biomarker selection using systems networks and decision theory
Supervisors: Mani Mudaliar - Target Analyst Director at Recursion | Arkaitz Zubiaga - Senior Lecturer and Deputy Director of Graduate Studies, School of Electrical Engineering and Computer Science, QMUL | Claudia Cabrera - Lecturer in Bioinformatics, Centre for Translational Bioinformatics, WHIR, QMUL
Yu-Yuan Yang
Yu-Yuan (Stuart) Yang, originating from Taiwan, is part of the second cohort of PhD students specializing in Artificial Intelligenc
e for Drug Discovery (AIDD). Holding a bachelor’s in Agricultural Chemistry and Chemical Engineering, as well as a master’s in Biomedical Electronics and Bioinformatics from National Taiwan University, Stuart brings over four years of extensive interdisciplinary research experience to the table.
His expertise spans agro-chemistry, protein engineering, bioinformatics, molecular dynamics, AI healthcare systems, and quantum informatics. Notably, he has contributed to a phage discovery project, applied projects on amyloid fibrils, developed genomics pipeline tools at the National Health Research Institute in Taiwan, and written a review on quantum algorithms for drug discovery. Stuart’s diverse skills are underpinned by a strong foundation in both wet and dry lab environments. Currently, he is delving into a structural biology project, working on a machine learning-based binding pocket classifier for drug design. For more about Stuart and his work, visit his personal website: Yu-Yuan (Stuart) Yang's Website (https://yuyuan871111.github.io/).
Project Title: Machine learning for structure-based drug & biologic discovery
Supervisors: Richard Pickersgill - Professor of Structural Biology and Head of School, School of Biological and Behavioural Sciences, QMUL | Arianna Fornili - Senior Lecturer in Computational Chemistry, School of Physical and Chemical Sciences, QMUL | Harikrishna Sekar Jayanthan - Senior Scientist, Evotec
Angela Abraham
Angela is a PhD candidate in the 2024 AI for Drug Discovery Programme cohort. She previously completed a BSc in Biochemistry with Biology at Keele University and an MSc in Human Molecular Genetics at Imperial College London, where her
interest in bioinformatics and data analysis began. She further developed her computational and research skills with over 2 years experience as a computational research assistant in genetic epidemiology at Brunel University of London and the University of Lincoln.
Her project aims to develop a high-confidence, directionally and functionally detailed model of protein interactions to better understand cellular pathways, predict disease mechanisms, and facilitate drug discovery.
Project Title: Developing a High-Quality PPI Interactome to Model Cell States and Disease Biology
Supervisors: Kirill Shkura - Systems Biologist and Data Scientist, MSD | Pedro Cutillas - Professor of Cell Signalling and Proteomics, Barts Cancer Institute, QMUL
Joy Efiannayi
Joy is a PhD student in the 2024 AI for Drug Discovery Programme cohort. She completed an integrated master’s degree in Chemistry with Biomedicine at King’s College London, and her final-year master’s project focused on the computational analysis of melanin for new material design.
Joy has a strong interest in leveraging computational tools to understand the complexities of cancer and develop AI-driven therapeutic strategies. Her current project builds on unique cancer driver detection by taking the next step toward identifying druggable targets specific to individual patients. By developing ML classifiers, Joy aims to advance personalized oncology by pinpointing new therapeutic opportunities, including potential drug targets and drug repurposing, that can lead to tailored and effective treatments for cancer patients. This work focuses on bringing precision medicine closer to clinical practice and improve outcomes in oncology.
Project Title: AI-based Identification of New Drug Targets for Personalised Oncology
Supervisors: Francesca Ciccarelli - Professor of Cancer Genomics, Barts Cancer Institute, QMUL and Principal Group Leader, Cancer Systems Biology, The Francis Crick Institute
Ivan Manoza
Ivan is a PhD student specializing in developing computational methods to reduce drug cardiac toxicity on ion channels, using physics-based molecular simulations and ma
chine learning. Ivan earned his bachelor’s degree in chemistry at Queen Mary University of London, where his final year project involved using molecular dynamics (MD) to investigate the cystic fibrosis transmembrane regulator, focusing on ion gating and permeation.
His research primarily focuses on the hERG ion channel, where drug-induced blockages pose significant concerns. He aims to predict the affinities and blocking propensities of new molecules, expanding beyond existing databases. In parallel, he is exploring blockers for the KNa1.1 potassium channel to address gain-of-function mutations linked to developmental and epileptic encephalopathies (DEEs). By combining advanced techniques, his work strives to broaden chemical space and enhance drug discovery efforts in the pharmaceutical industry.
Project Title: Reducing Drug Cardiac Toxicity Using Molecular Simulations and Machine Learning
Supervisors: Vytautas Gapsys - Principal Scientist, Janssen Pharmaceutical | Wojciech Kopec - Lecturer in Computational Pharmaceutical Chemistry, School of Physical and Chemical Sciences, QMUL
Syed Zayyan Masud
Hello! I’m Zayyan, a PhD student in AI-driven drug discovery (AIDD) focusing on G-Protein Coupled Receptors (GPCRs) and Graph Machine Learning. I hold a degr
ee in Pharmacology from Queen Mary University of London (QMUL), where I first developed my interest in GPCRs. I later explored computational drug discovery techniques targeting GPCRs during my MPhil at the University of Cambridge, which led me back to QMUL for my PhD.
My research focuses on leveraging graph-based machine learning to identify GPCRs as potential drug targets. GPCRs are ubiquitous membrane proteins involved in a wide range of physiological functions, and nearly 30% of currently marketed drugs target these receptors. My work explores using knowledge graphs to analyse signalling data related to GPCRs and their roles in various diseases.
Project Title: AI-driven Omics Data Integration and Transfer Learning for Target and Biomarker Identification
Supervisors: Hermanus Draisma - Senior Scientist, Translational Biomarker Discovery & Bioinformatics at Nxera Pharma | Conrad Bessant - Professor of Bioinformatics, School for Biological and Behavioural Sciences, QMUL
Constance Maurer
Constance Maurer is a PhD student in the AI for Drug Discovery Programme, conducting her thesis at Queen Mary’s Barts C
ancer Institute (BCI) in Dr. Angus Cameron’s laboratory. Her project focuses on the integration of CRISPR screens and artificial intelligence to explore the interactions between cancer cells and the tumour microenvironment (TME), with the aim of identifying novel therapeutic strategies.
Having started her career as a molecular biologist specialising in developmental and rare diseases, Constance's work was grounded in translational medicine after completing her MSc in Genetics of Human Disease at University College London. In 2020, she transitioned into data science and bioinformatics, merging her molecular expertise with computational techniques.
Now, she is applying these combined skills to cancer biology, developing innovative methodologies to model and target the complex interactions within the TME. She aims to integrate large-scale CRISPR screen, proteomic, and transcriptomic datasets, applying machine learning to identify potential drug targets that can modulate the immune-suppressive microenvironment and impede tumour progression. Her work highlights the potential of AI-driven approaches to accelerate drug discovery and elucidate molecular and cellular mechanisms supporting cancer progression. Ultimately it is hoped this work will bring new therapeutic strategies to the clinic.
Project Title: Combining CRISPR-screens and AI to Identify Novel Therapeutic Strategies to Target Cancer-Stroma Interactions
Supervisors: Otto Morris - Senior Biological Data Scientist at Exscientia | Angus Cameron - Reader in Cell Signalling and Tumour Biology; Director of Graduate Studies, Barts Cancer Institute, QMUL | Pedro Cutillas - Professor of Cell Signalling and Proteomics, Barts Cancer Institute, QMUL
Marta Niedostatek
Marta Niedostatek is a PhD student on the AI for Drug Discovery Programme and also part of the School of Mathem
atical Sciences. She has an MSci in Mathematics from King’s College London. Her research interests are in network science with a focus on higher-order networks and their application in biology and medicine.
Project Title: Higher-order network inference for prediction of gut microbiome-cancer interaction
Supervisors: Ginestra Bianconi - Professor of Applied Mathematics, School of Mathematical Sciences, QMUL | Nicola Perra - Reader in Applied Mathematics, School of Mathematical Sciences, QMUL